In October 2019, Google announced the BERT Algorithm.
The update affected 10% of all search queries.
Then there was Google's Core Update in January 2020:
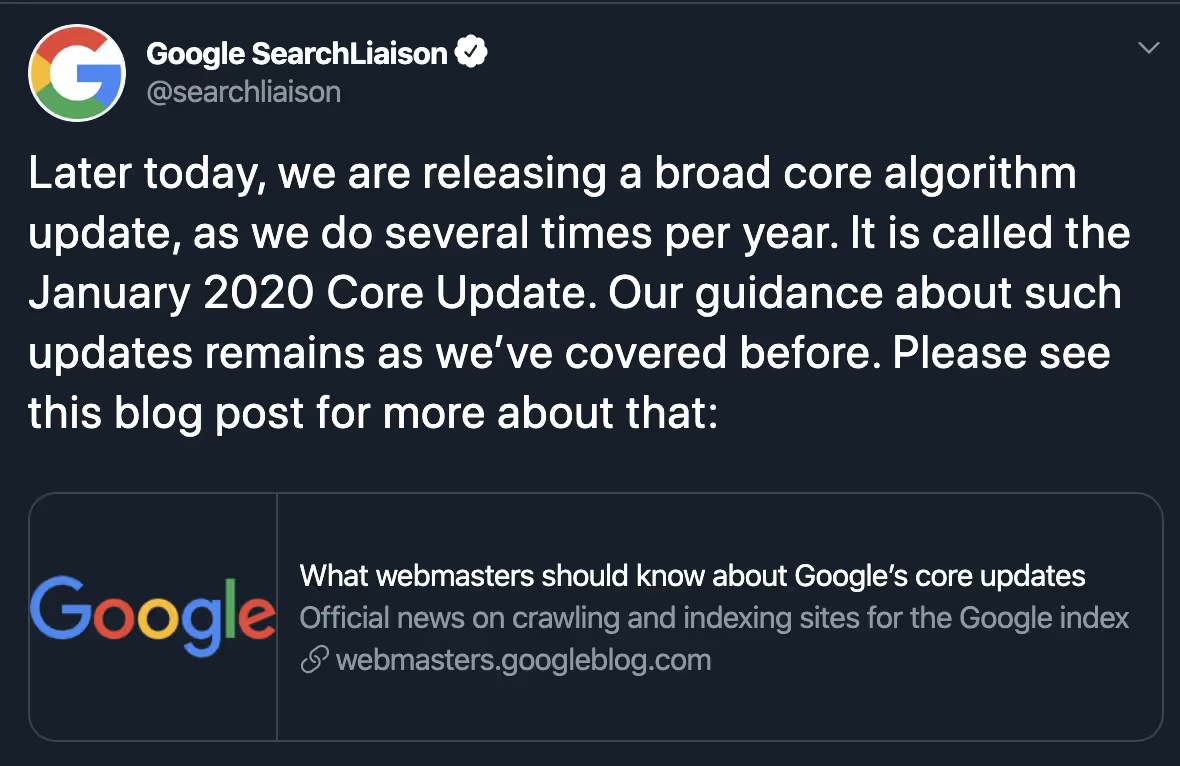
The tweet refers to a blog post about Google's core updates from August 1st, 2019.
Recently, we saw activity in SERPs, and SEOs have to determine what it means for search engine optimization.
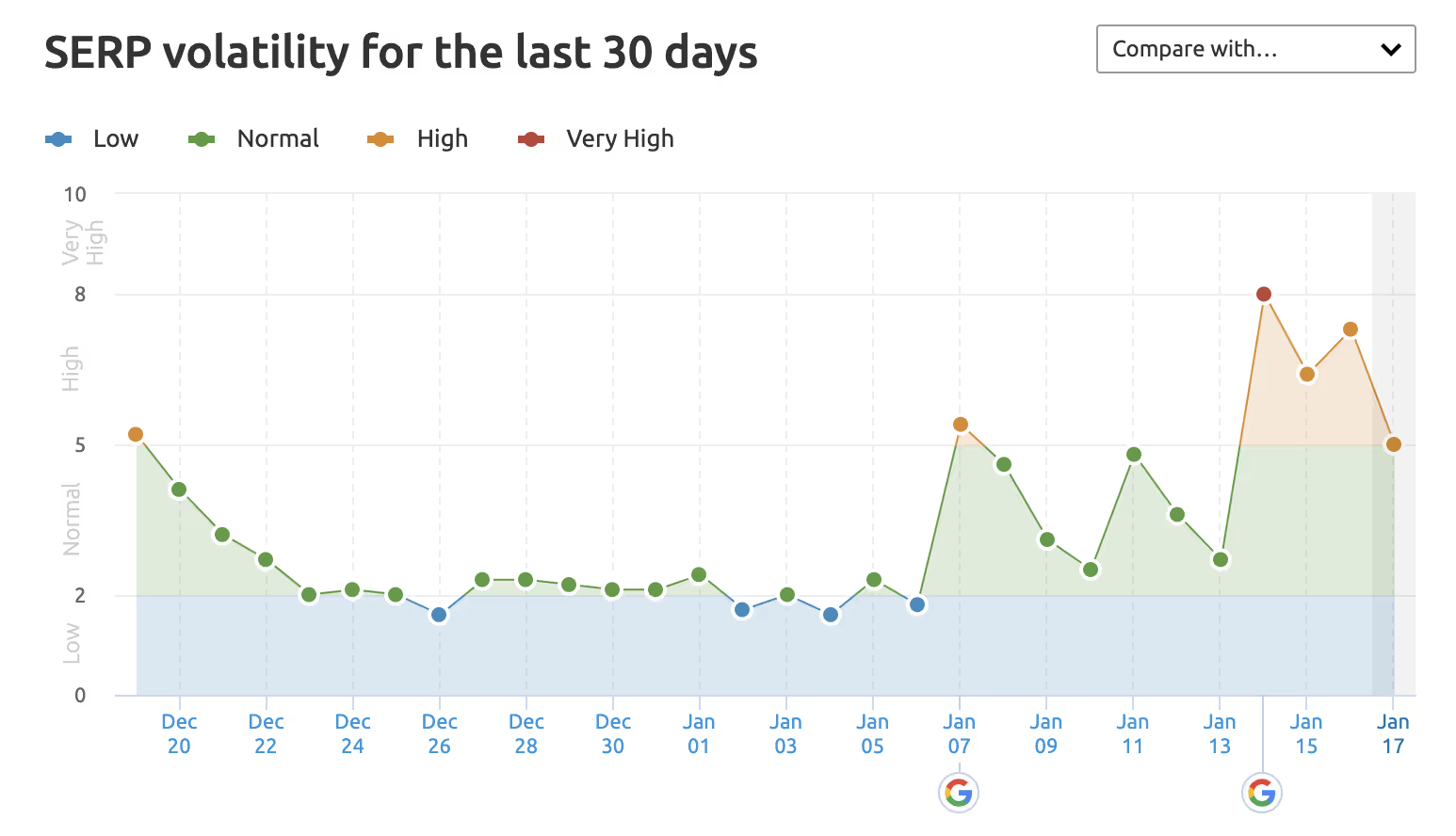
When changes happen, our SEO process needs adjusting to keep up with the search engine algorithms.
BERT brought the rise of quality content, context, modern machine learning, and natural language processing (NLP) is undeniable.
What's behind all those buzzwords?
Fascinated by BERT and NLP, I spent hours checking tools, analyzing datasets, and testing solutions. I put my findings in one article so you know what it means for you, your website, and your clients' site.
Spoiler alert: there is a way to implement NLP SEO as an enhancement to your search engine optimization, and how you use it to optimize your website. I included some case studies at the end so that it all makes sense.
BERT is the key to understanding the NLP concept, and the latest change in Google to factor into your SEO strategy.
Why do BERT and NLP appear in one sentence so often?
In October 2019, Google announced an update that allows bots to understand the context and search intent and reflect the changes in search results.
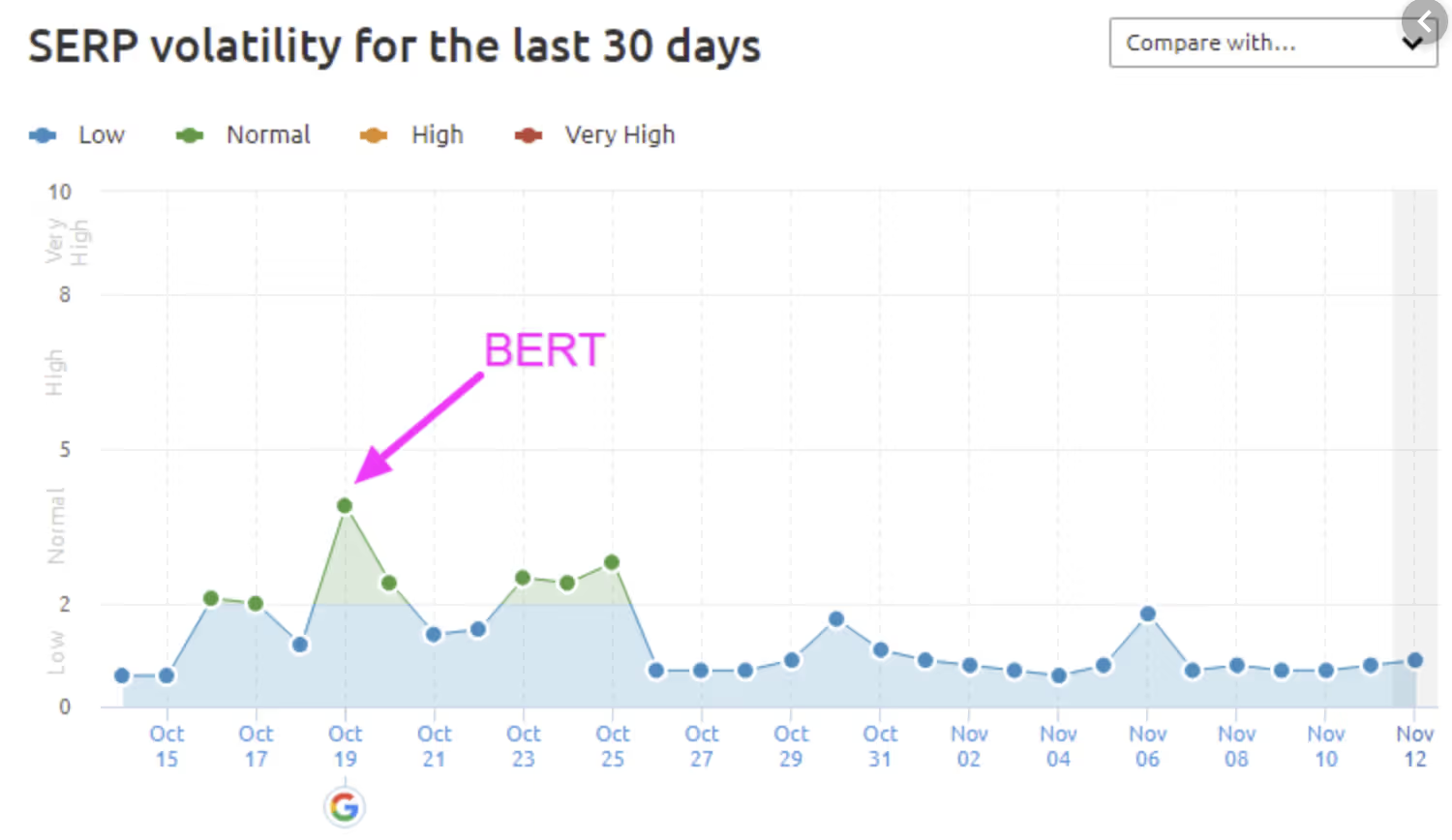
According to Google:
These improvements are oriented around improving language understanding, particularly for more natural language/conversational queries, as BERT is able to help Search better understand the nuance and context of words in Searches and better match those queries with helpful results.
BERT stands for Bidirectional Encoder Representations from Transformers. The first part, “Bidirectional,” is crucial to comprehend NLP methodology—especially since we can find at least two explanations of "bidirectional" that make sense.
The first definition states, that “bidirectional”, itself, refers to two directions of a process.
Basically, Google finds the meaning of a word or phrase using both.
The other definition refers to learning ability.
BERT evaluates the meaning from a context (first direction) and demonstrates learning ability and natural language understanding (second direction). We believe the process is completely unsupervised.
Both explanations help to understand the NLP process and its effect on search engine optimization.
The relationship between BERT and NLP
BERT contains two major components: data (pre-trained models) and methodology (defined way to learn and use those models).
Models are essentially sets of data, but you need a way to process these datasets. Without a process to correctly interpret them, they're useless.
BERT is an essential part of NLP and affects search engine results.
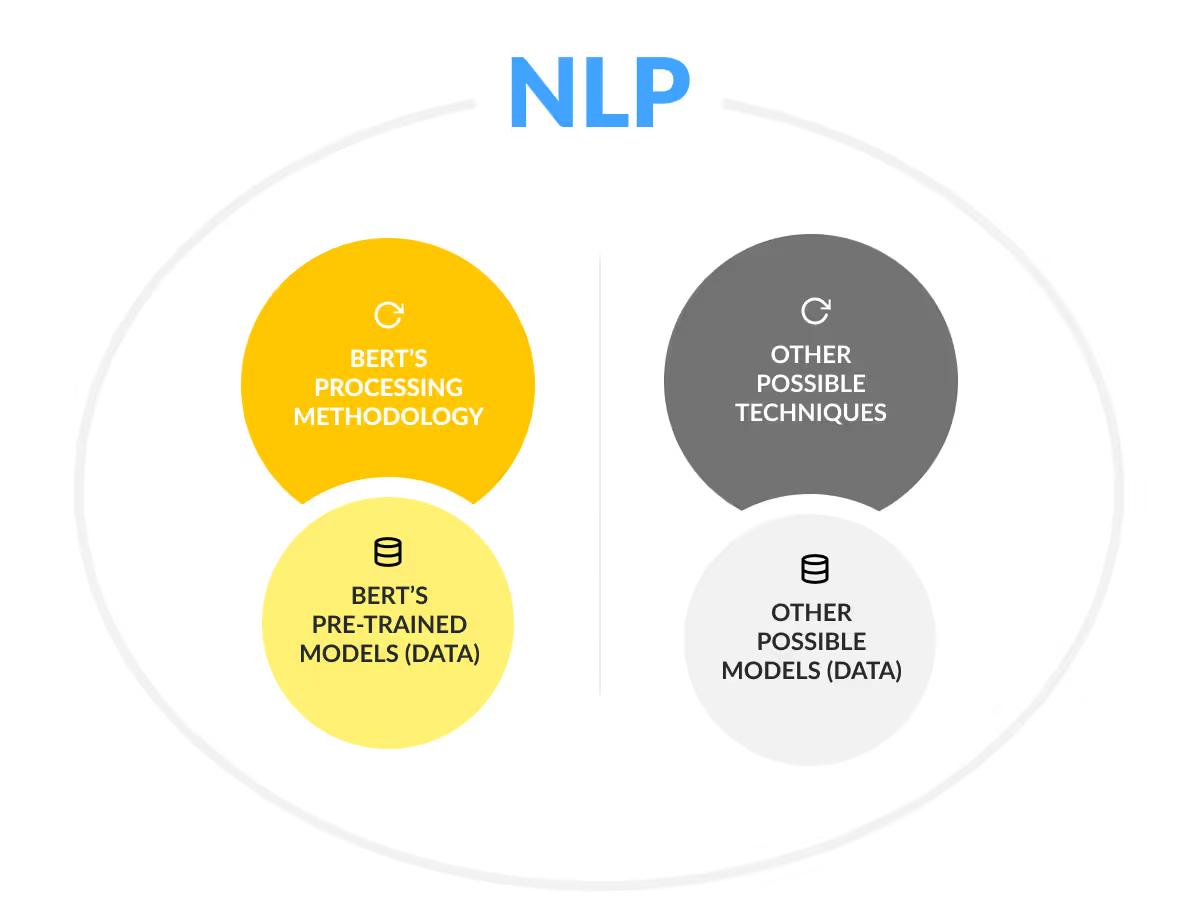
More about the history of NLP: Sean Shuter published an in-depth article that covers this.
How do BERT and NLP impact SEO?
In one word: SIGNIFICANTLY!
Since Google’s algorithm uses NLP, it impacts on-page and off-page SEO.
But to be more precise, NLP changes the way we understand queries as a whole, and each word separately. Besides that, Google is capable of assessing the sentiment of selected entities from the website’s content.
Practical examples of BERT's impact on featured snippets
At Surfer, we have the privilege to work with loads of data, I took advantage of the data I have access to and analyzed it with BERT.
As Google stated, they changed the way of understanding search queries.
So, I selected five thousand English phrases and compared SERPs from before and after.
The results confirmed that from an SEO perspective, BERT was impactful.
After the update, the number of queries with featured snippets grew by 5.2%!
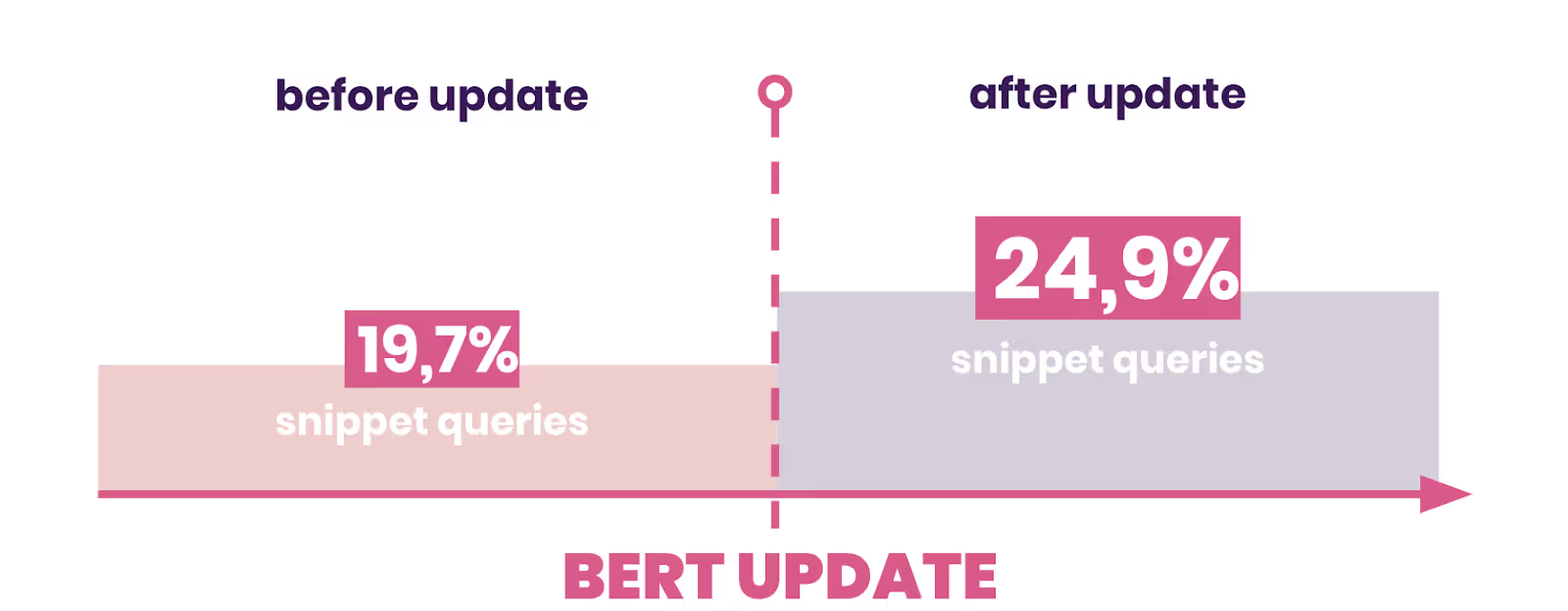
How does NLP enhance the search quality?
According to Google's blog, 15% of search queries are used for the first time. People use their search engines for long tail searches to find answers to questions especially with the rise of voice search.
Sometimes the algorithm doesn't have enough historical data to anticipate the intent behind the search term, thus it has difficulties delivering relevant search results.
Essential to any keyword search; the language; either spoken (voice search), or written.
To quote the statement from Pandu Nayak's article, which we consider to be a trustworthy source:
With the latest advancements from our research team in the science of language understanding--made possible by machine learning-- we're making a significant improvement to how we understand queries, representing the biggest leap forward in the past five years, and one of the biggest leaps forward in the history of Search.
Context is everything
For more sophisticated and conversational queries, it's hard to assess the meaning of prepositions.
Many queries contain high-frequency words like "and", "to", "in". There are also words with multiple meanings like "get", "go." Let's look at an example.
The expression "go" can be meaningless, but take a look at what happens in different contexts.

Examples from Google’s blog:
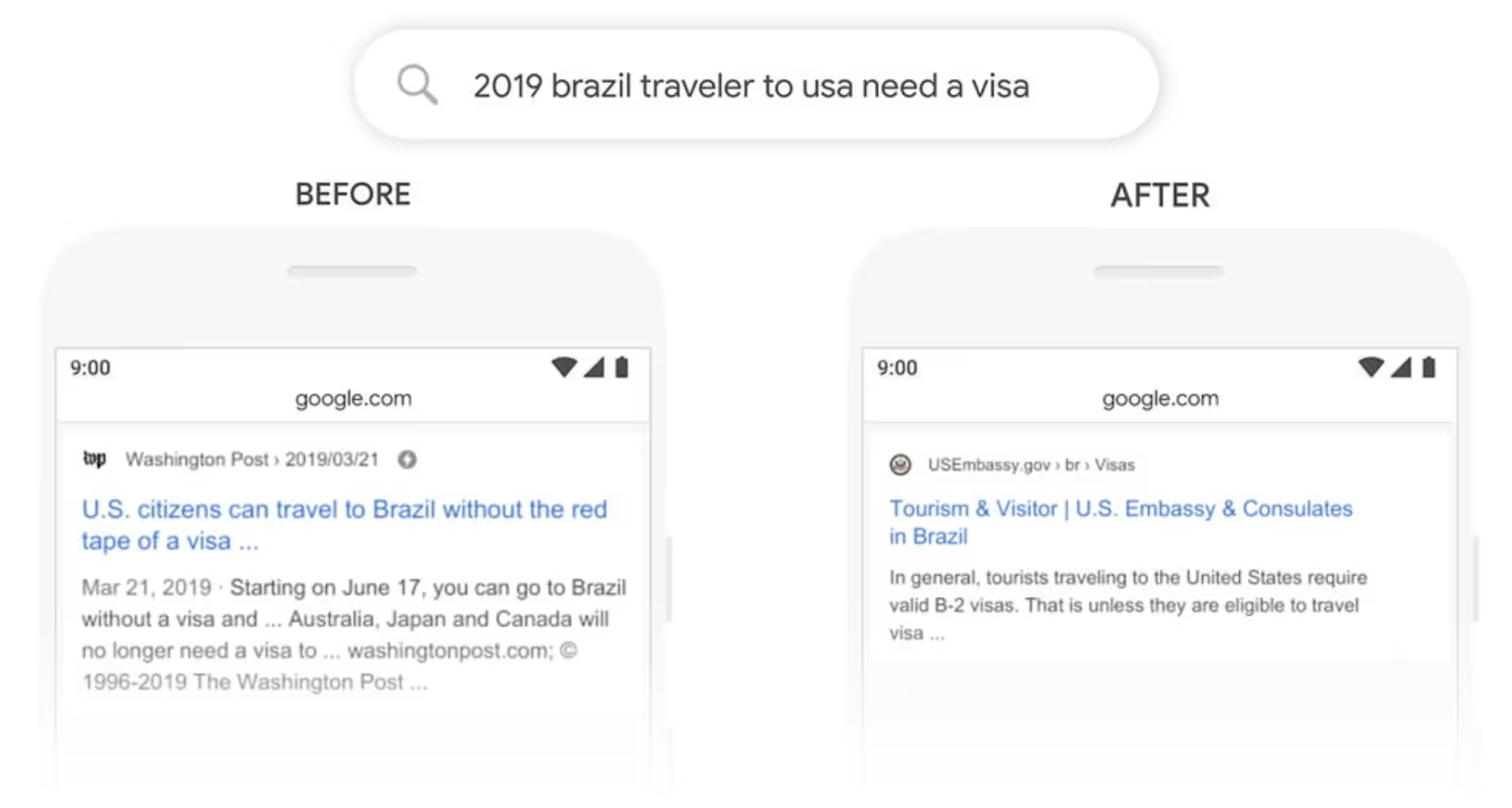
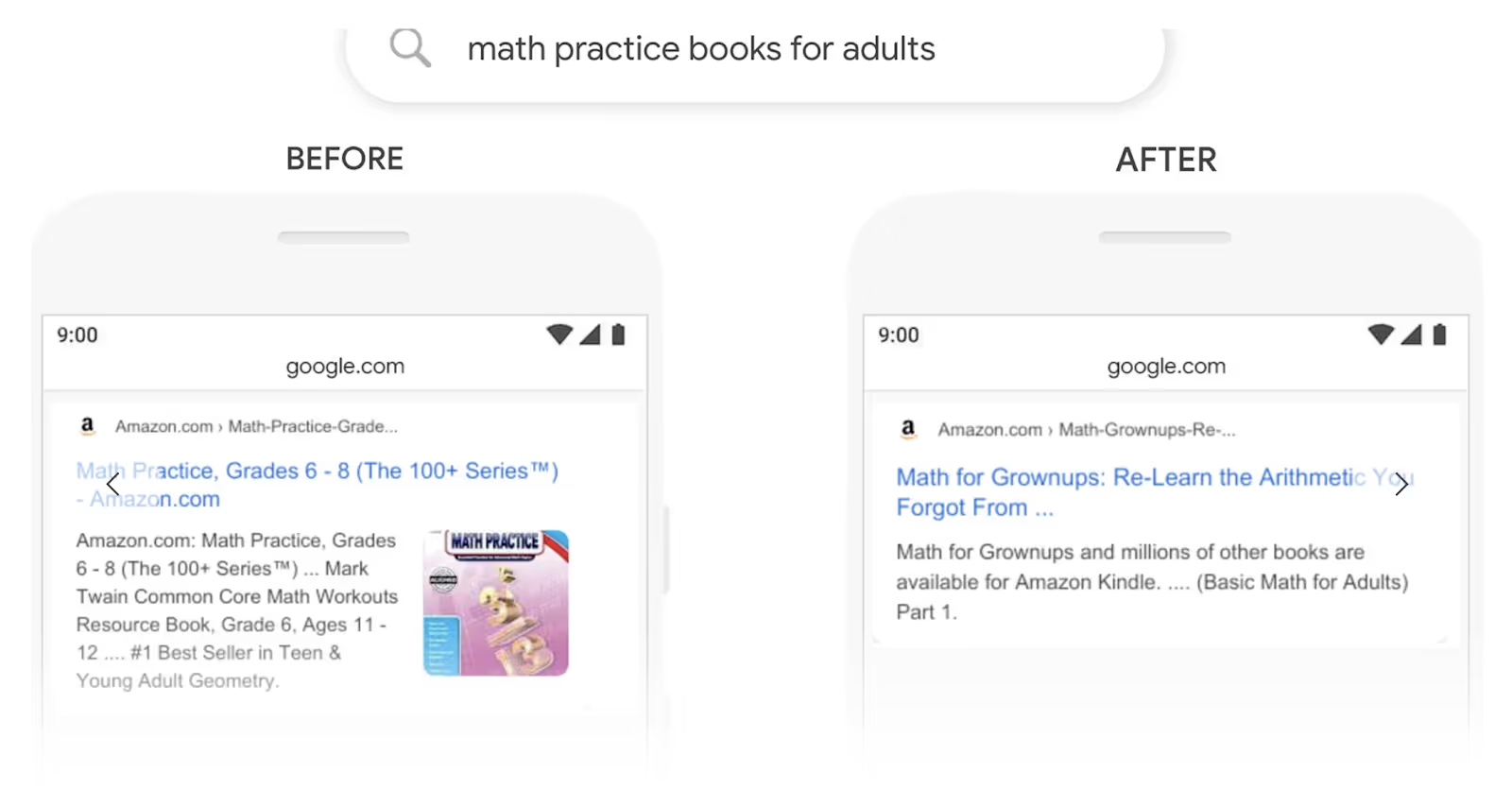
What is sentiment in NLP?
The sentiment is the undertone. The sentiment can be positive, negative, or neutral.
Positive sentiment means the topic is being described favorably. They usually have positive words like “great”, “guru”, “hero”, or “outstanding.” The sentiment is positive if the value of it is between 0.25 – 1.0.
Negative sentiment suggests the usage of detrimental statements in the content. As you probably have already guessed, those pages use words like “hate”, “weak”, “stubborn”, “boring”, “danger”. Negative sentiment: -1.0 – -0.25.
Finally, neutral can contain both positive and negative signals, and the value is -0.25 – 0.25.
Remember: Google's algorithm calculates the sentiment value not only for the entire subpage, but for each subsection of the content.
Does sentiment affect position in SERP? Read our recent study about NLP sentiment analysis in SEO.

What is entity in NLP?
Entity is a word or phrase that represents an object which can be identified, classified, and categorized.
Objects are persons, consumer goods, events, numbers, or organizations. NLP's job is to select and evaluate the entities.
Since Google distinguishes those entities, the search engine utilizes information to satisfy the user and provide better search results.
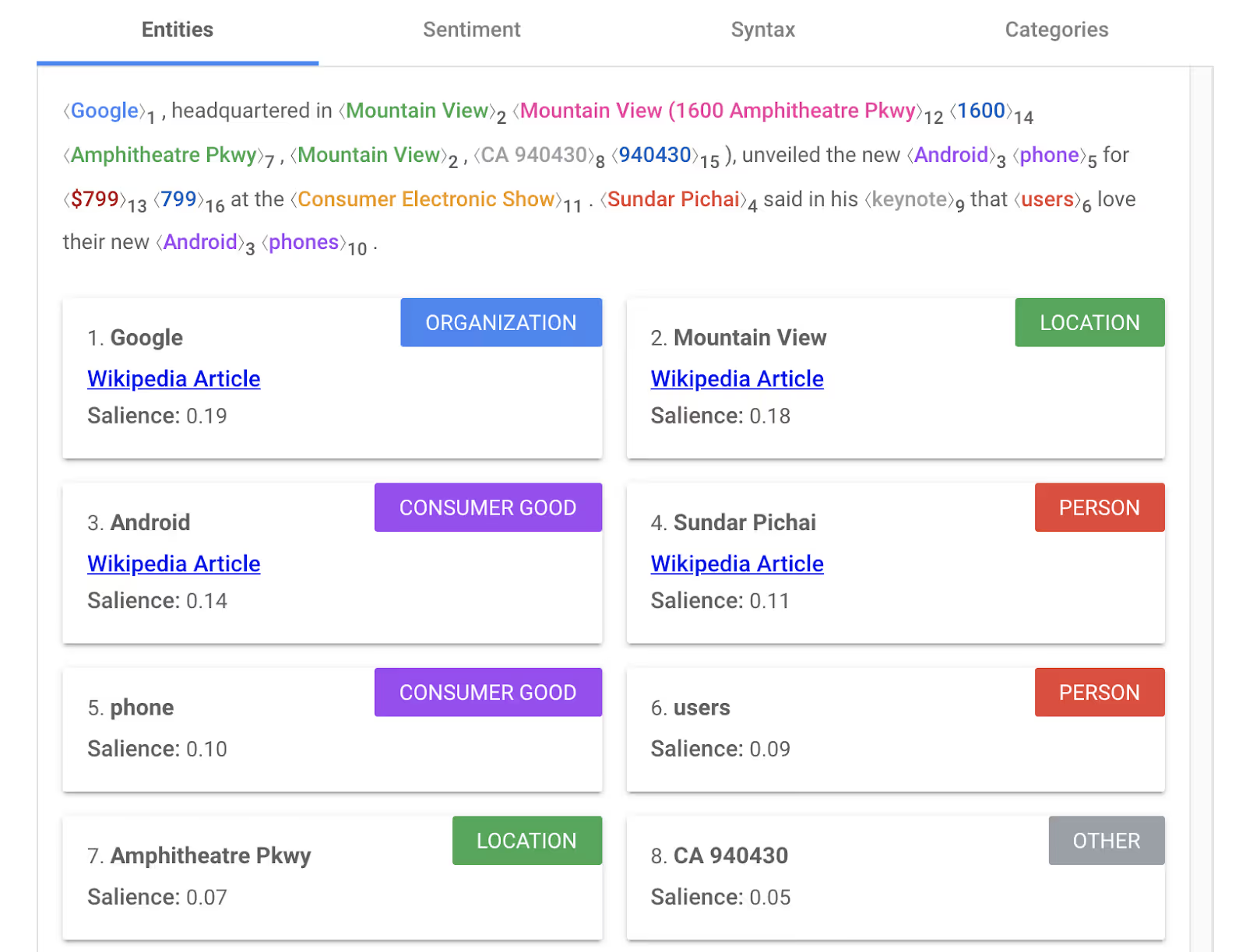
Two additional metrics: salience and category.
Thanks to NLP, Google is capable of assigning the content to a corresponding category, such as /Internet & Telecom/Mobile & Wireless, in the following example.
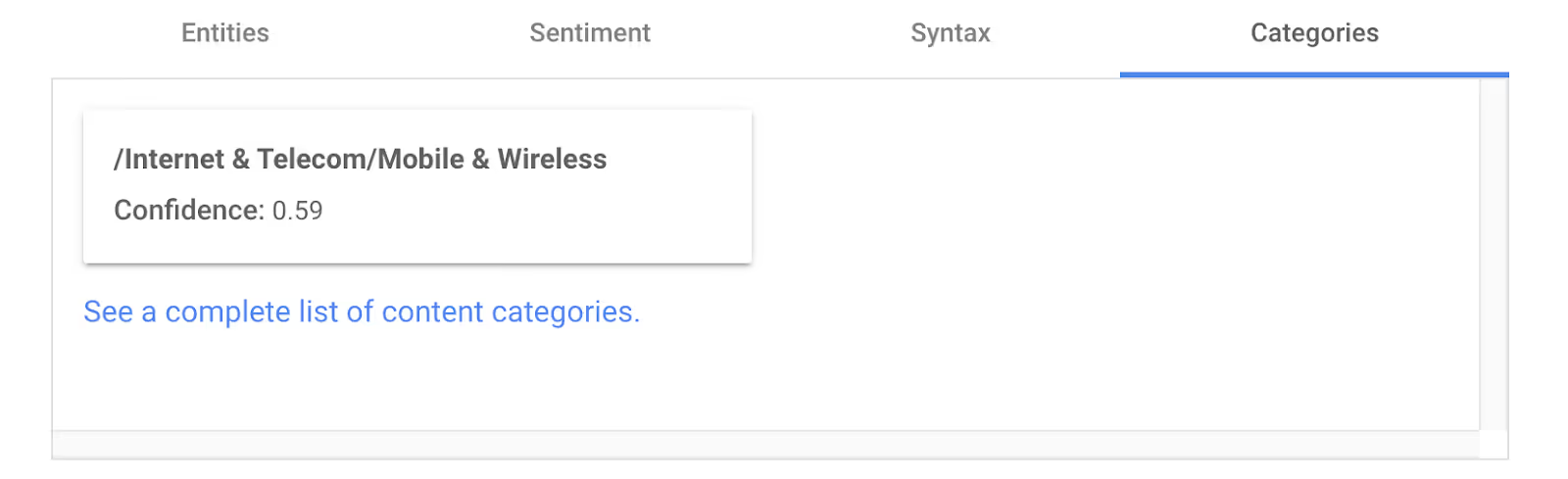
Check out the complete list of content categories.
What is salience in NLP?
The salience in NLP represents the entity's importance in the text. The range is from 0.0 to 1.0. The higher salience value, the more relevant the entity is for the subject of the page.
For example, the word “morning” may be more important than “evening” when we talk about breakfast.
Use Google's Natural Language API demo
The first step to enhance the SEO process is to use the available tools. The most important is Google's Natural Language API demo, a free tool.
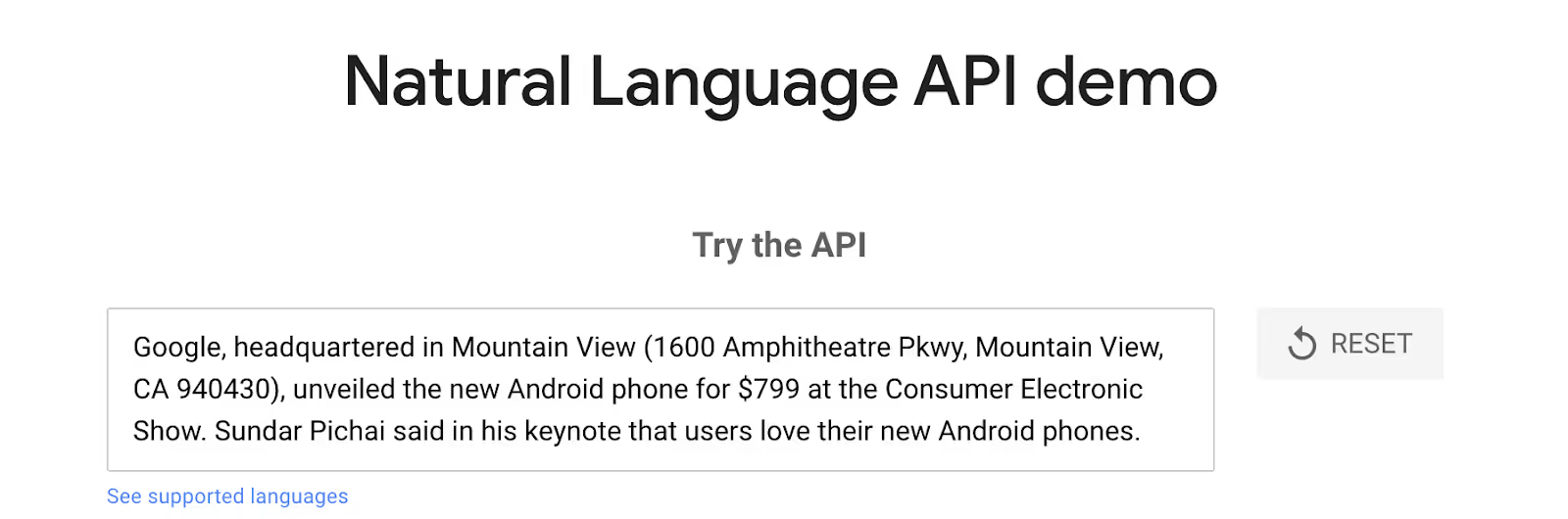
There are a few limitations: it doesn't support every language. Here's a NLP supported languages list.
How to optimize your website for BERT?
After Danny Sullivan's tweet, I've read about BERT and NLP, the objection is that there is nothing you can do to optimize your website for NLP.
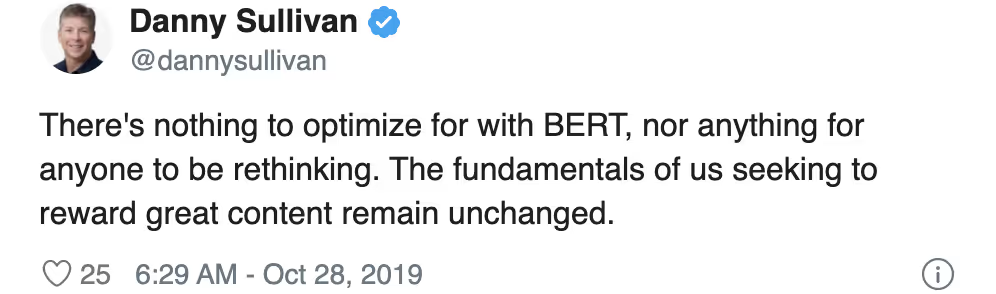
During one of the more recent Google's webinars, John Mueller's was asked what kind of SEO work we could do regarding BERT.
He explained that:
(...)the queries are not really something that you can influence that much as an SEO (...)the text on the page is something that you can influence. Our recommendation there is essentially to write naturally(...)."
He mentioned special attributes we need to watch out for, and said it can be helpful to "match the query that someone is asking with the specific page."
Writers write in a way humans can understand; not focusing on keyword stuffing, but natural language.
Steps to boost your SEO results:
Keyword research: use Google Search Console to find lost rankings
As Google understands each query much better after the BERT update, there were noticeable fluctuations in SERPs.
It's important to diagnose what types of queries increased their traffic and select the ones that noted the loss in organic search traffic.
Some pages may have shown a loss in organic traffic. In many cases, Google's algorithm expects a different type of content. Your content doesn't seem to be relevant, but decreases in traffic are not necessarily a signal that your website is no longer interesting for people.
To find out which keywords suffered from the drops post-update, use Google Search Console.
Select the keywords that dropped in rankings, and compare your content to the current competitors. Maybe it needs to be rewritten, or the topic's coverage isn't complimentary. Maybe your content is too long, or lacks the proper seo entities?
Detect keywords to take care of right away thanks to GSC. Here's how in two steps:
Step #1
Open GSC, click date filter and compare October 2019 to November 2019 as BERT hit at the end of October.
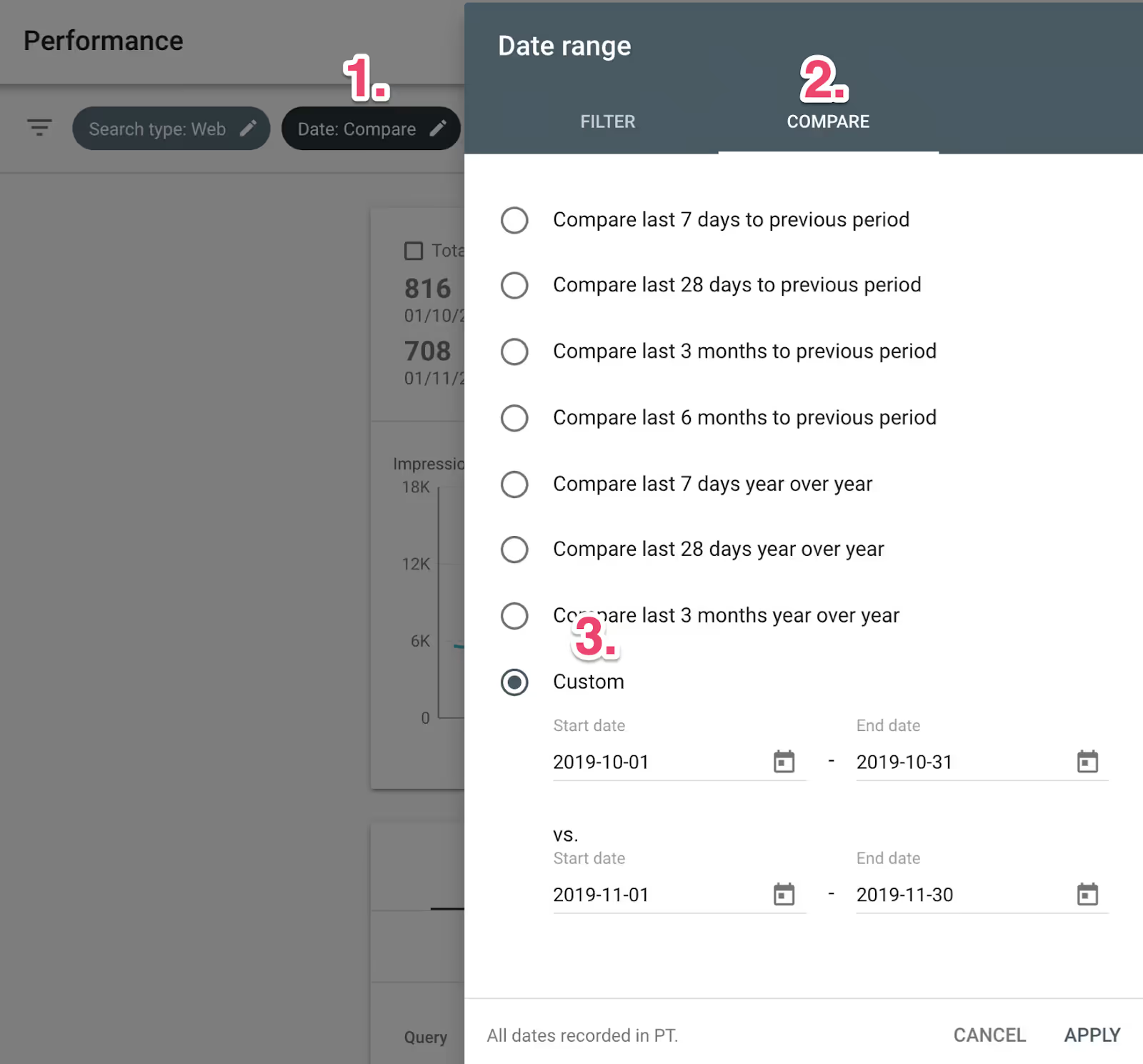
Step #2
Pick impressions and sort by difference. Shuffle between Queries and Pages to find out if a single page or set of pages got hit.
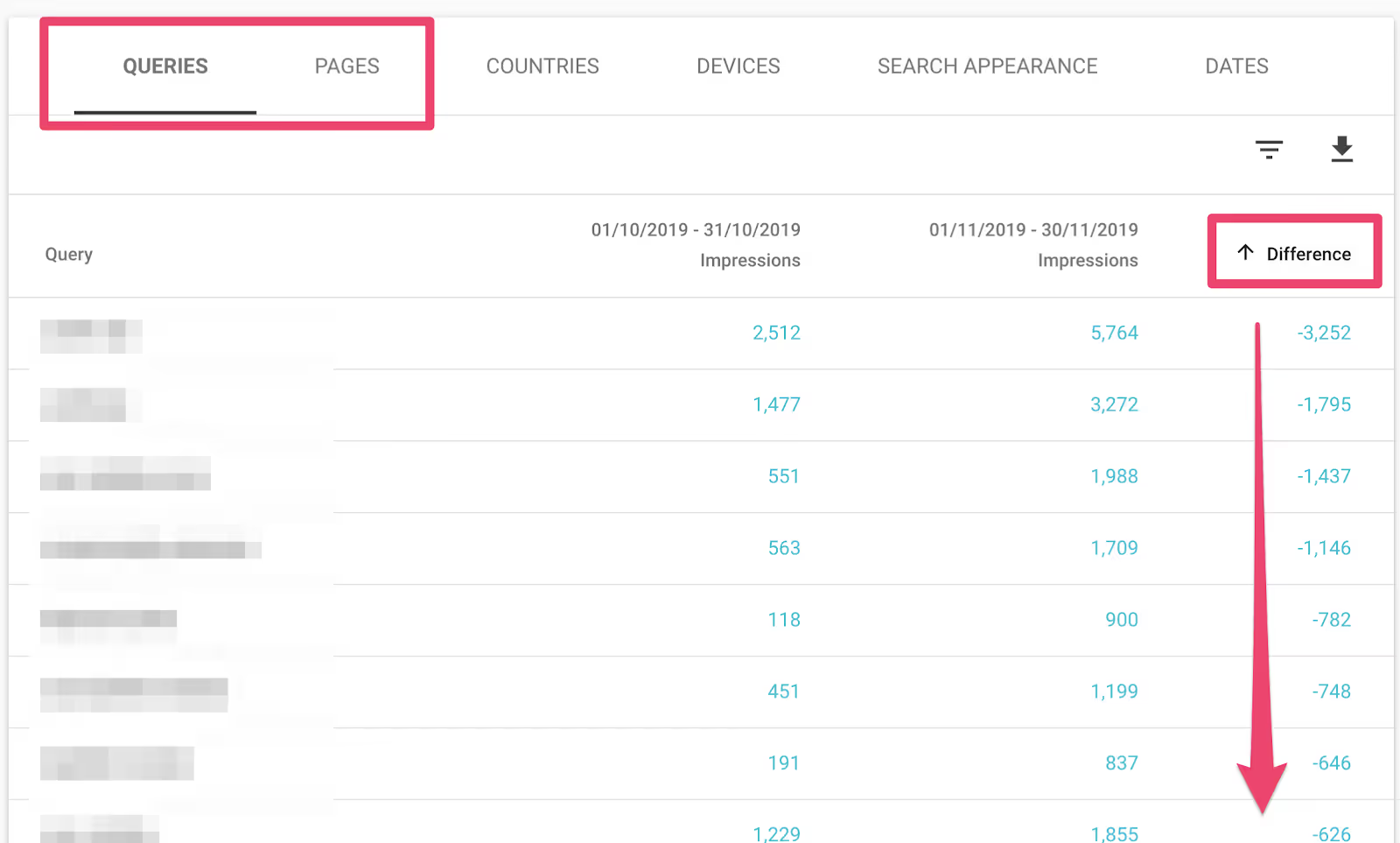
Now you have prioritized keywords and pages list to take care of.
Restructure the website
Search algorithm expert from Bertey, Dawn Anderson, explains:
There will still be lots of work for us to do since we need to emphasize the importance, utilize clear structures, help to turn unstructured data into semi-structured data, utilize cues on content light pages (e.g. image-heavy but not text-heavy eCommerce pages) using such things as internal linking.
In some cases, the internal linking and website structure plays a significant role in the process of understanding the content.
It means if your website is poorly written or doesn't have a clear structure, it may get lost on the 2nd page. I would consider "the structure" regarding the whole domain as much as a single subpage.
Optimizing the website's structure:
- Taking care of internal links,
- Internal anchor text unification,
- Usage of the comprehensive navigation,
- In some cases, breadcrumbs implementation.
Optimizing the single article entails:
- A proper schema implementation,
- The keyword stuffing elimination,
- Improvement of headers' structure,
- Providing the sources of data and author,
- Taking care about topic's coverage by comparing against best competitors,
- Include proper entities related to the topic,
- Upholding preferred sentiment not only for the entire content but for each entity as well, etc.
Take a look at our article on SEO for blogs for tips about bringing organic traffic in.
Take care of internal and external backlinks
Thanks to NLP, Google's algorithm evaluates the context of internal and external links. For now, the links structure and placement are more important than ever before.
Both internal and external backlinks are one of the most substantial SEO factors. BERT is used to redefine a backlinks' profile as well as an internal website's architecture.
A link placed in the right context has higher value than a randomly placed one. So your links, internal or external, bring you more juice if:
- Placed on a website with a niche connected to yours,
- Placed on a page that has a similar context,
- Placed in a paragraph that is logically related to the content of your page.
Hunt for snippets
There are new opportunities within search engines to adjust the SEO process to the new reality: snippets.
Take the advantage of search engine optimization and use snippets to provide answers for FAQs.
Competitors' analysis—SEO process with NLP factors
After analyzing any text written in the supported language (through using Google's NLP API), you'll get metrics, including:
- Sentiment,
- Entities,
- Category,
- Salience score.
Step #1: Select your main keyword
Choose your primary keyword you'd like to rank.
Keep in mind that some keywords are competitive. In our article on keyword difficulty evaluation, we answered the question on how to pick a query you can realistically rank for.
Step #2: Select your competitors
It may seem obvious, but many SEO experts think every website listed in the top ten is a page they compete with. This kind of approach may dilute your dataset.
To select them properly you need to:
- Define your content type. Even if you're going for a “research” search intent, you need to know if you're writing a blog post, creating a video, or a landing page.
- Exclude pages that serve different intent.
- Exclude outliers: pages that are much longer or much shorter than other ones.
- Exclude pages that rank because of their authority and backlink profile.
Here is an in-depth article about picking the right SEO competitors for your analysis.
Step #3: Create a document to gather the data
To collect data in a comprehensive form, I'd recommend using a spreadsheet, text document, or whatever works for you best. Here is one of my templates for NLP competitors' analysis. It's downloadable, so you can use it for your own purposes.
Download the spreadsheet here.
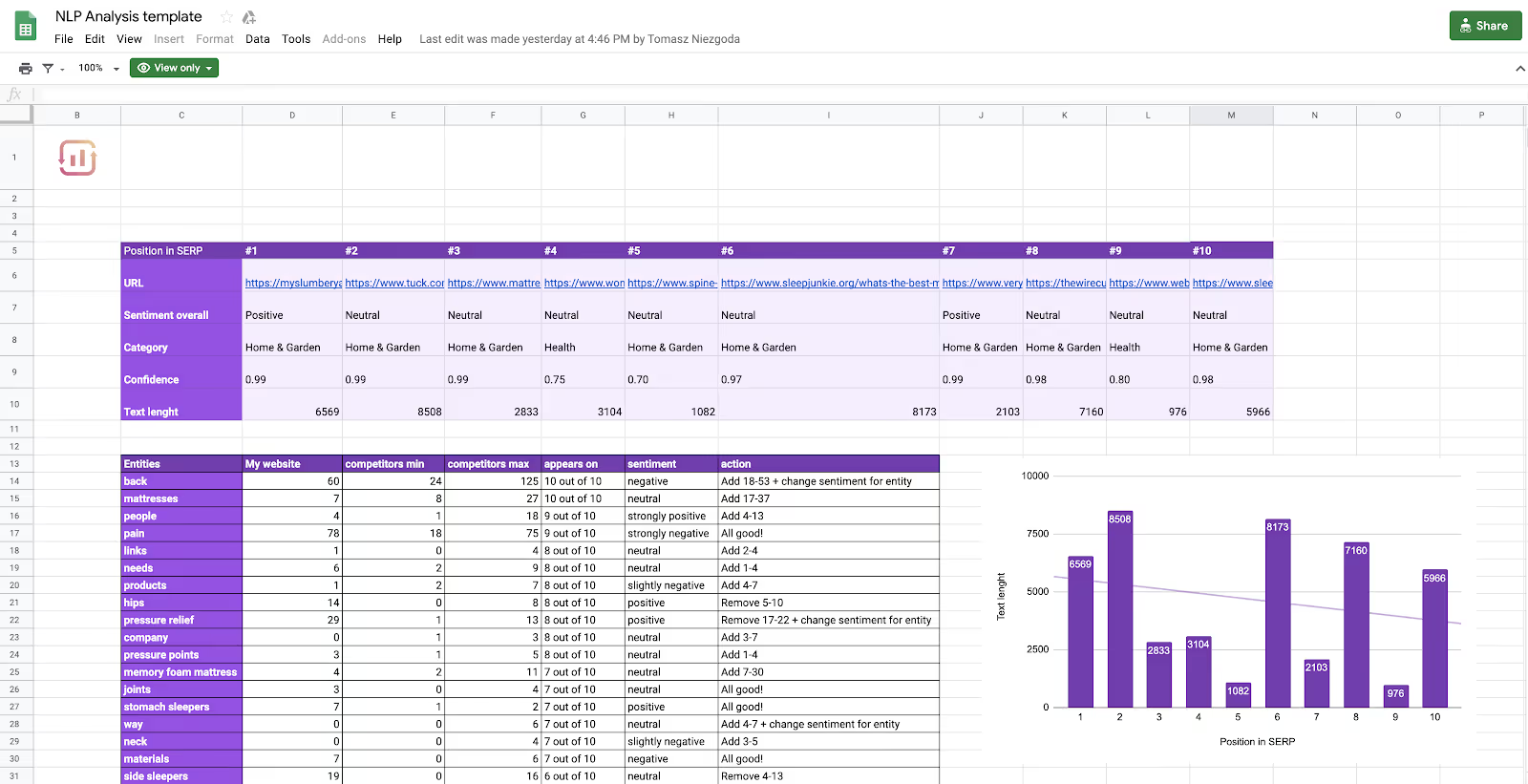
Step #4: Compare your content to your competitors
Especially in case of one-sided SERPs, be aware of using the preferred sentiment. Going for the opposite might make all your SEO efforts in vain.
An easy example would be if all top search results of a product with positive reviews, and you created a negative review, that might negatively impact your rankings. Google has taken the historical data of the more relevant sites and apparently it favors positive reviews over the negative ones.
Even though Google experiments with the sentiment, and it fluctuates over time, it can be challenging to get into the top ten with an unexpected undertone. Similarly, sometimes it is impossible to overtake one of the highest organic positions in Google with the content short on entities' appearance.
Comparing yourself with direct competitors allows us to fill the gap, estimate the user's intent behind the search query, and make the content more comprehensive.
How well does Google assess sentiment?
As much as I love the entities' selection, the sentiment valuation leaves room for improvement.
I analyzed a bunch of keywords to find a one-sided negative SERP.
It was tougher than I expected.
Although in most cases there are positive and neutral sentiments, Google is very reluctant to assign the negative sentiment to websites.
On the other hand, we have to keep in mind that BERT is just one of the pre-trained models. We don't have any confirmation that it's the same version that is used by Google's algorithm. I assume that Google doesn't have much interest in sharing the newest version of their pre-trained models with us. After all, why share the best-performing resources?
Let's take a look at an example that shows sentiment analysis for very negative content.
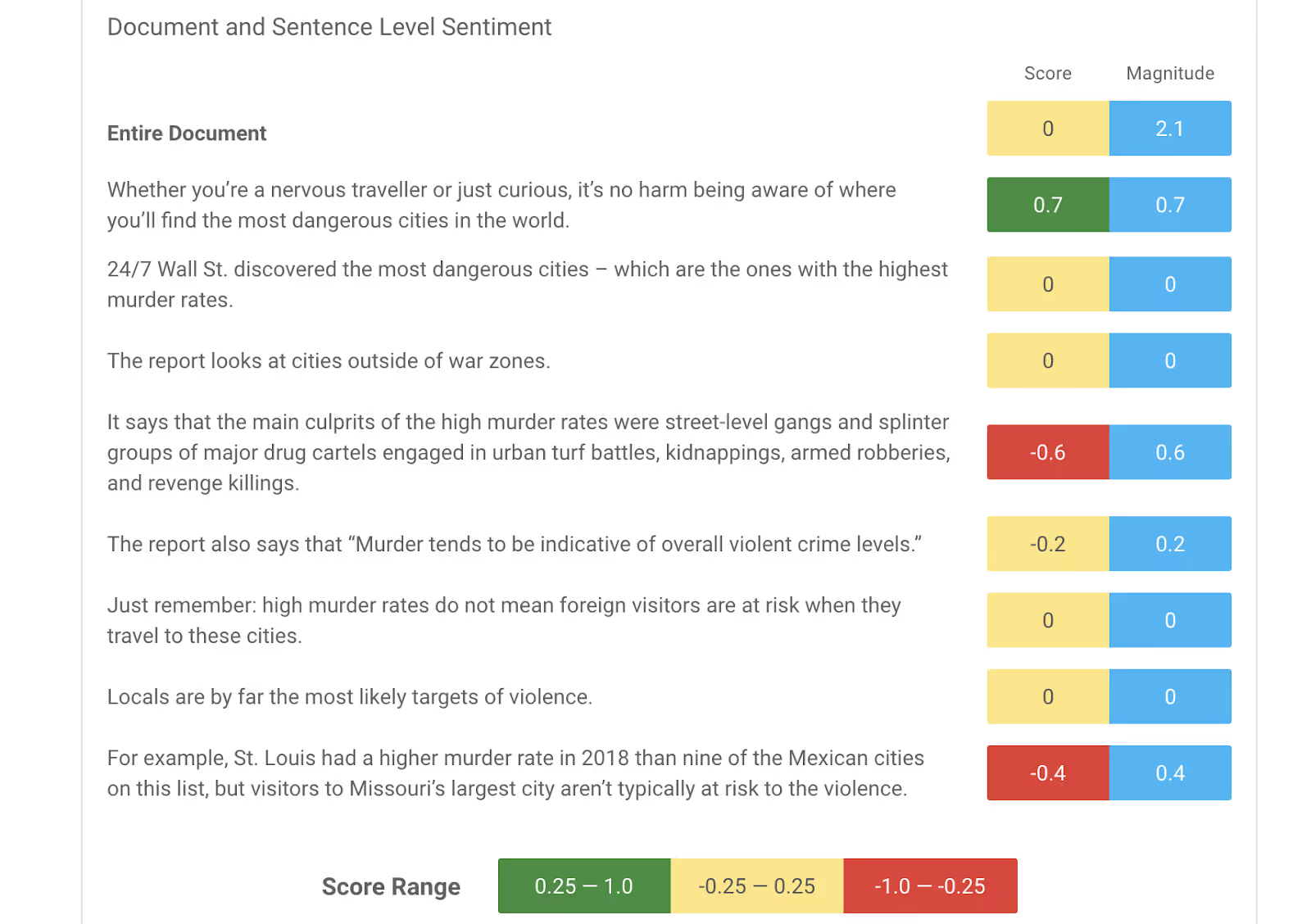
BERT alternatives for sentiment analysis
BERT is just one of the NLP models. Moreover, Google isn't the only company that develops NLP techniques. BERT's alternatives are:
- Watson (IBM)
- ULMFiT
- Transformer
- Transformer-XL
- OpenAI's GPT-2
IBM Watson sentiment analysis
I tested IBM's Watson. It's pretty amazing and performs better than Google and it gained some popularity in SEO communities. I observed that Watson recognizes negative statements much better than Google.
This time, I tested SERPs to find a correlation in the sentiment value.
I discovered that for less technically advanced users might come across technical difficulties.
Sending API requests directly from the Macbook terminal requires basic technical knowledge.
The request must be preceded by generating your unique API key, etc. In return, we get the data in a particular order.
Here is the fragment of the response for the same piece of content about the dangerous cities that I used for Google API example.
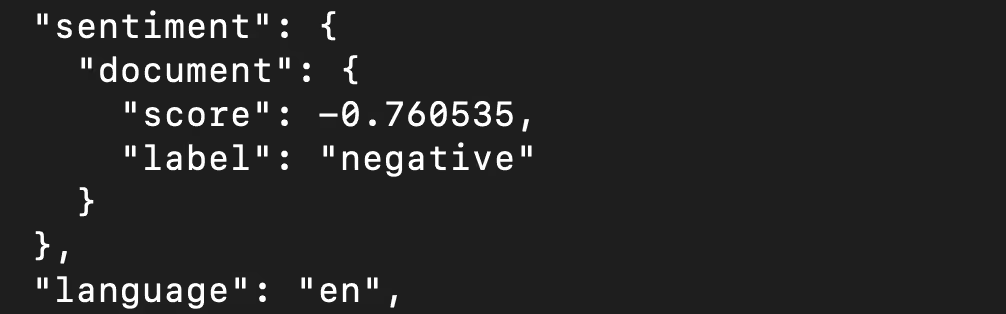
Technical knowledge isn't necessary for data interpretation. For Watson, the same piece of content is strongly negative, whereas Google's BERT sees it as neutral or even positive.
Now let's compare the top 50 on the chart forkKeyword: "most dangerous cities in the world 2019" in the United States. The chart below presents the NLP analysis according to Google and to IBM Watson Sentiment.
At Surfer, we decided to use both Google (for True Density entities and entities sentiment) and IBM Watson (for the general sentiment). The NLP Analysis will be released soon.
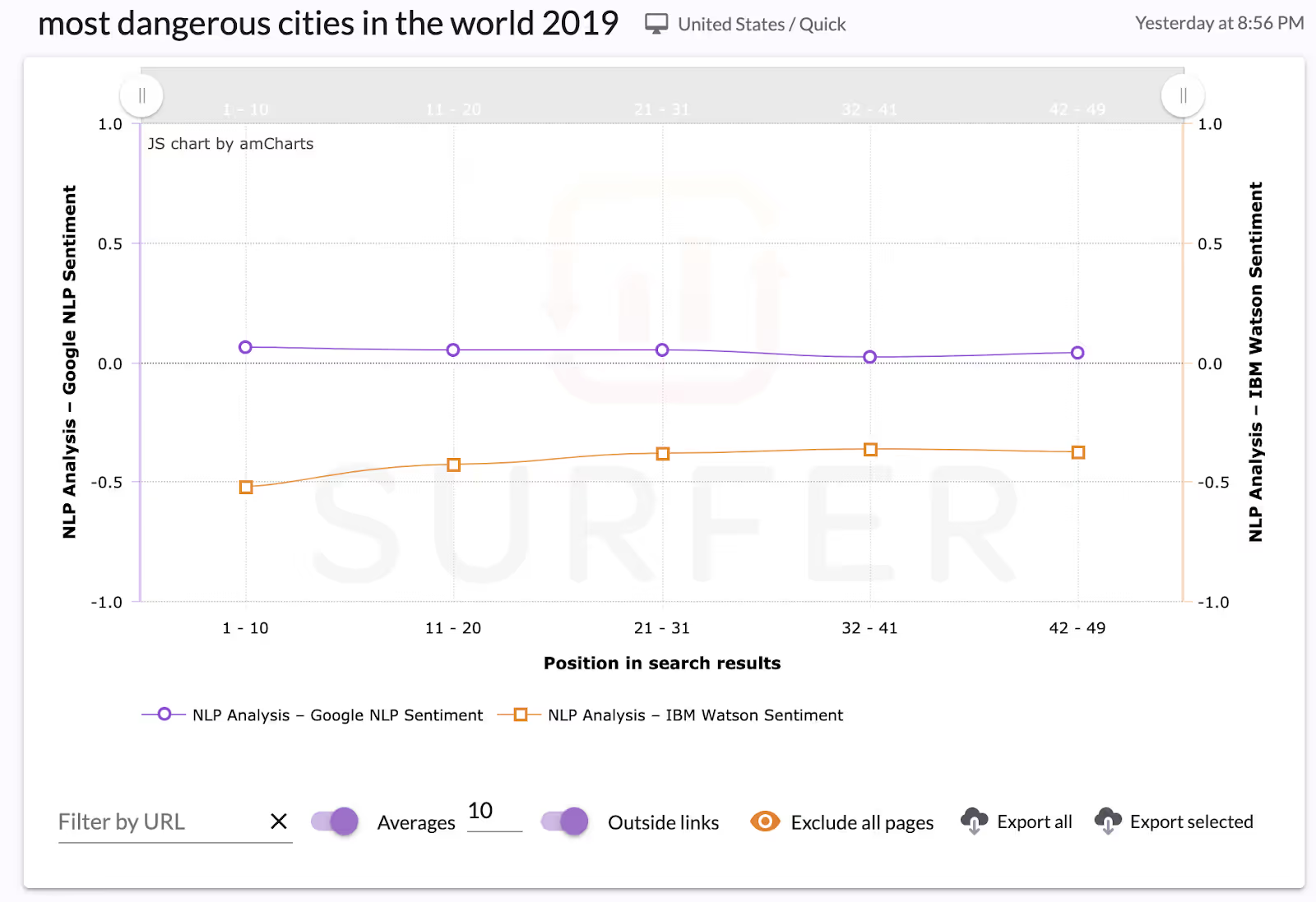
BM’s Watson and Google’s NLP sentiment analysis comparison
There is a sentiment correlation in the analysis conducted by Watson. At the same time, there is no correlation in Google's NLP chart.
Correlation is not a causation and it's always good to remind ourselves of that, but Watson's negative sentiment assessment looks more probable.
Note that I compared a single piece of content with an averaged sentiment score. I know that some of you disagree with this kind of approach, because:
- IBM's Watson may use a completely different methodology than Google's NLP.
- It's more important to put entities in a proper vicinity than keep a whole article in particular sentiment.
Even though both statements shed some new light on content, there is no evidence that Watson's sentiment assessment is or isn't credible. I have tested many queries and Watson looks much more reliable.
Using entities in the right sentiment neighborhood seems to be fundamental when it comes to NLP. But for less demanding queries I would advise you to write naturally for humans and don't pay attention to such details. However, a granulated entities' sentiment report can be very helpful with the most competitive queries.
Watson's NLP can be applied in many different cases—IBM generates a meaningful income out of providing API access, and there is no reason to hide a well-performing solution.
NLP and SEO case studies update
As I said at the beginning, we have conducted a bunch of tests around NLP. One of them was held during the January 2020 Core Update.
You all know that the best tests are done on real-life use cases. I asked my teammate, Michał Suski, to encourage a few of Surfer's users to create the NLP audit. Then he implemented entity-related tweaks on their pages and the results were interesting.
The analyzed article was already ranking quite well. (primary terms on the bottom of the first page), so I didn't want to do a huge overhaul of the page.
1. Colin Ma, Digital Entrepreneur & SEO of Nimble Made
I added almost all of the terms Surfer NLP Analysis suggested, but I added a little less for the first page update. For example, Surfer suggested adding the word “dress” 5 times and I added it just 2 or 3 times.
I didn't want to change the content too much since, in this case, it was clearly doing quite well already. Overall, the additional entities allowed me to add in some extra keywords by allowing me to see product names, and names of other items related to the article.
I was impressed by the large boost I got from the NLP optimization as I moved up 5-6 spots on page 1 within 2 days.

2. John Pinedo, the CEO of Freedom Bound Business
The Surfer team wasn't lying when they said the new NLP-enabled Surfer beta would be a game-changer.
I had a keyword stuck at position 5-6 for months! A week after running an audit (NLP entities enabled) my target keyword, along with the secondary keywords, moved up in the SERP.
Aside from better rankings, I really like the new NLP column that's included in the audit. It shows examples of how competitors use phrases/words without having to go to their page which just makes optimizing for true density a lot easier!
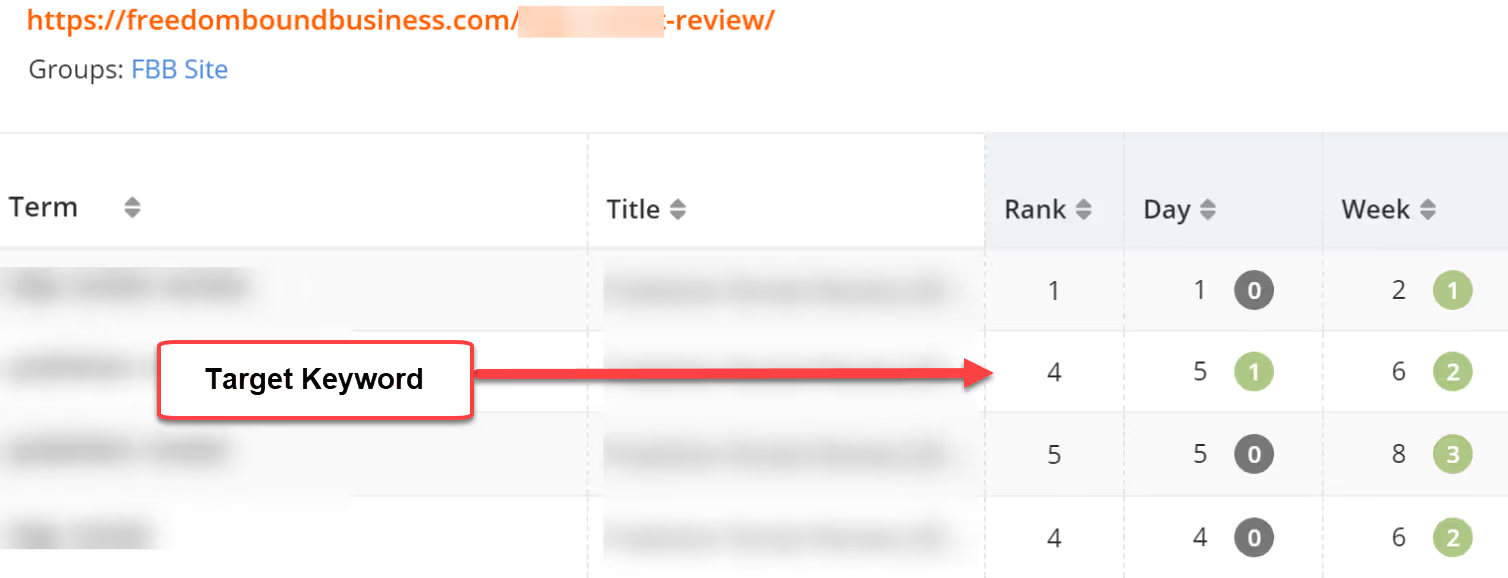
3. Matt Diggity of diggitymarketing.com
A small teaser of ranking boost on one of the blogposts from Matt Diggity. Pure NLP optimization using Content Editor.
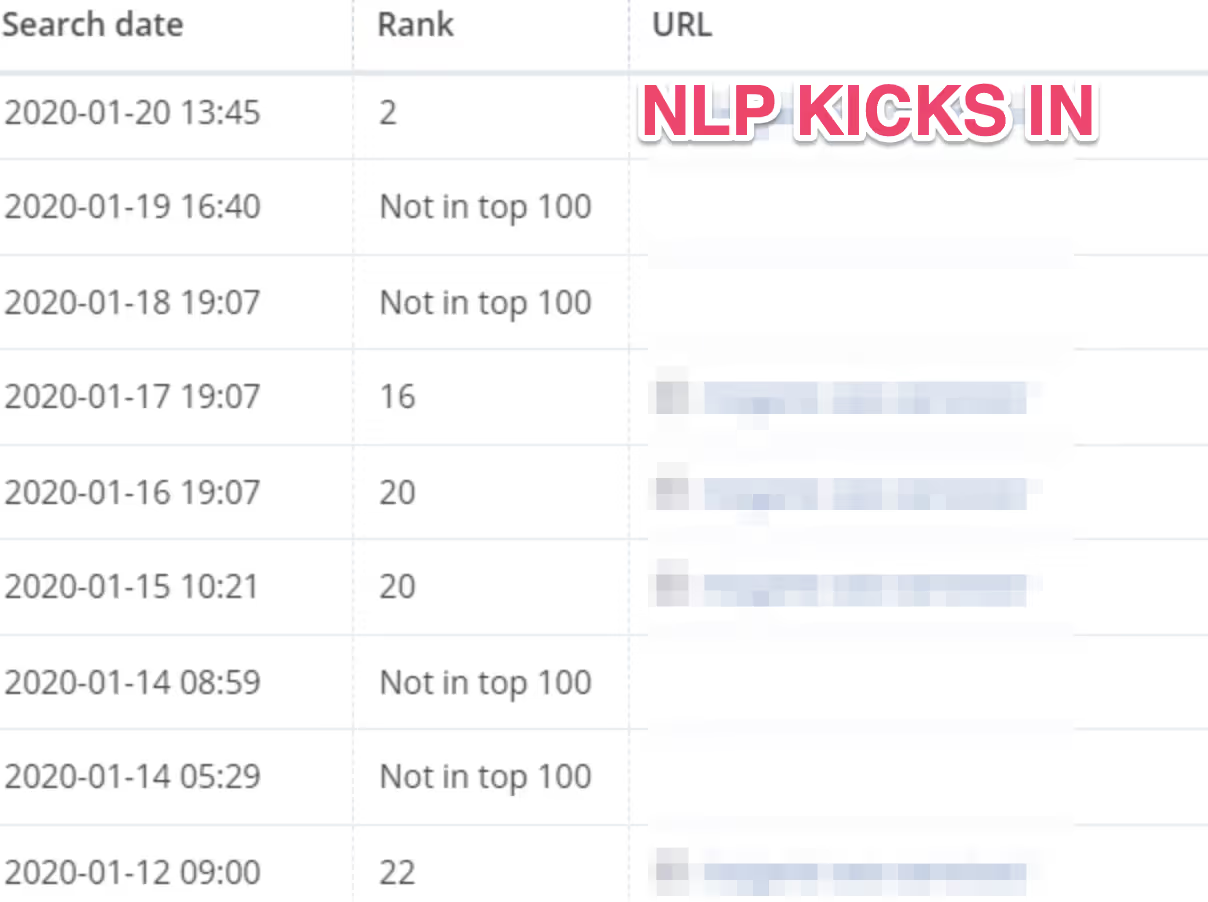
Summary
NLP is a very complex concept. As an SEO specialist, you don't need to be a machine learning expert to use available tools to enhance the SEO methodology. Let the search engines' tools do the hard work for you.
But having some basic knowledge, which is confirmed by SEO tests, and observing SERP fluctuations will help you to keep up with the industry. Still, if you'd like to find out more about NLP, Future Processing has recently written a great post.
In short, here's how to NLP-optimize your website for success:
👉 Restructure your website and pages 👉 Analyze the sentiment 👉 Add relevant entities you're missing
I'm curious about your tests' results and position changes caused by the BERT Update. Have you already included natural language processing components in your analysis? Are you using NLP analysis when conducting deep SEO Audits? Share your adventures with the update!